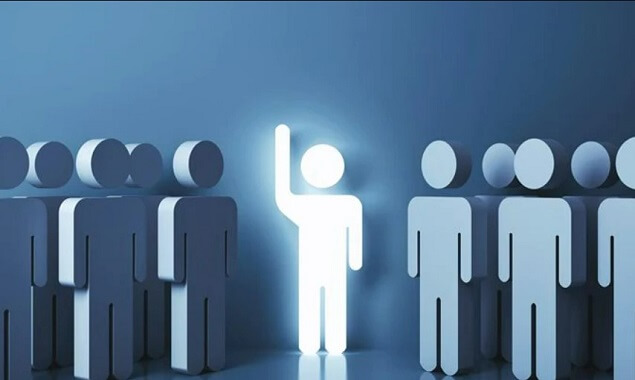
16 Haz Generative AI and the future of HR
A chatbot may not take your job—but it will almost certainly change it. Here’s how to start thinking about putting gen AI to work for you.
Generative AI: It’s powerful. It’s accessible. And it’s poised to change the way we work. On this episode of the McKinsey Talks Talent podcast, talent leaders Bryan Hancock and Bill Schaninger talk with McKinsey Technology Council chair Lareina Yee and global editorial director Lucia Rahilly about the promise and pitfalls of using gen AI in HR—from recruiting to performance management to chatbot-enabled professional growth. An edited version of their discussion follows.
What’s so different—and so disruptive
Lucia Rahilly: There has been so much buzz in recent months about generative AI and tools like ChatGPT. Many people seem to be ricocheting between wonder at the potential of these tools and fear of their inherent risks. Lareina, what’s different about generative AI, and what’s behind its disruptive potential?
Lareina Yee: A couple of things stand out about generative AI. In November 2022, OpenAI released ChatGPT 3.5, and within five days, there were a million users. So the speed of adoption was unlike anything we’ve seen.
For me, what was most profound about that moment was that anyone—of any age, any education level, any country—could go onto GPT, query a question or two, and find something practical or fun, like a poem or an essay. There was an experience there that was accessible to everybody. We’ve seen a lot of advancement in the technology since then, and it’s only been a couple of months.
A second super-interesting thing is you don’t need to be a computer scientist to leverage the technology—it can be used in all types of jobs. OpenAI’s research estimates that 80 percent of jobs can incorporate generative AI technology and capabilities into activities that happen today in work. That is a profound impact on talent and jobs, and it’s different than how we’ve talked about it before.
In some ways, the genie is out of the bottle. It’s probably not the best strategy to try to put it back in. Lean forward and figure out how to use it in a way that’s productive and safe.
Lucia Rahilly: The immediacy of the use cases feels so novel and so lightning fast. Explain what generative AI is, so we’re working from a common definition of that term.
Lareina Yee: Generative AI is a technology that prompts the next best answer. A lot of people have used ChatGPT to summarize information, to draft a response to something, by pulling together an enormous amount of public data. But there’s also amazing imaging. I might want a song, audio, video, or code. Code is a huge example. It’s amazing the range of things that generative AI can do in the world, and it’s just getting started.
Bryan Hancock: I asked ChatGPT about myself, and it accurately reported that I do a lot of work on talent. However, it inaccurately reported that I went to Cornell because it assumed that Cornell was the most appropriate answer based on my background instead of the University of Virginia—where I did go. I thought it was very interesting that you don’t necessarily get what’s right but rather what’s logical.
Lareina Yee: In some ways, that emulates how we think. I’m not suggesting it’s thinking the way humans do, but in many ways, we use shortcuts and cues to make assumptions. That is kind of why people say, “Gosh, it feels really clever.” But to your point, Bryan, it’s not 100 percent accurate. There’s a great term for that: “hallucinating.”
What gen AI means for recruiters . . .
Lucia Rahilly: We’ll talk more about some of the risks, but let’s turn to what these kinds of generative AI capabilities mean for talent in particular. Do you expect generative AI to reshape or alter the recruiting process in any meaningful way?
Bryan Hancock: I think it’ll reshape recruiting in two meaningful ways. The first is helping managers write better job requirements. Generative technology can actually pull on the skills that are required to be successful in the job. That’s not to say managers don’t need to check the end product. They’ll need to be that human in the loop to make sure the job requirement is a good one. But gen AI can dramatically improve speed and quality.
The other application in recruiting is candidate personalization. Right now, if you’re an organization with tens of thousands of applicants, you may or may not have super customized ways of reaching out to the people who have applied. With generative AI, you can include much more personalization about the candidate, the job, and what other jobs may be available if there’s a reason the applicant isn’t a fit. All those things are made immensely easier and faster through generative AI.
Bill Schaninger: The best application of gen AI is in large skill pools where you’re trying to fill a reasonably well-known job. We need a more productive and efficient way to navigate all the profiles coming through. Where it makes me a little anxious is anytime it’s a novel job—a new role—or even, in US law, a job that’s changed more than 25 percent or 33 percent. In those cases, you have to go back and revalidate the criterion by which you would judge people in or out of the pool.
The challenge with validation is you need a performance criterion to regress against and say, “What’s the difference?” In some cases, that means figuring out how to get that criterion out of a data lake without encroaching on other people’s proprietary performance data. If you say, “Well, we’re only going to use our data as the employer,” then you are only basing the criterion off people you’ve already hired. And to validate, you have to look at the people you didn’t hire.
So it doesn’t mean the technology can’t be used. It just means there’s probably a little bit more front-end work on applying it to novel jobs and a wide-open opportunity for the big skill pools.
Lucia Rahilly: We talk a lot about having over-indexed on credentials and under-indexed on skills in the recruiting process. Does generative AI have a role in accelerating that shift from credentials like college degrees to the skills that candidates are actually capable of contributing to the workplace?
Lareina Yee: I’m optimistic it can. One thing this technology does extremely well is tagging—the ability to tag unstructured data for words. There are a lot of businesses that are thinking about applying that to e-commerce, to different types of retail experiences. But you could also apply it to talent acquisition or looking for capabilities. Now you don’t need to look for a credential or a degree. You could look for keywords in terms of capabilities and skills.
Looking at social media, how do people talk about certain capabilities? You may find there are better words to associate with those who have those skills. Think of a world where you want to be able to find candidates who have amazing experience from learning on the job but don’t have PhDs or college degrees. I’m optimistic that this could open more doors for folks like that.
Bill Schaninger: This is an interesting trade-off in the business world, which likes proprietary data sets and grouping of profiles. The real power might be, “How much can you get in the public domain until you start bumping up against paywalls?”
Long ago, when LinkedIn was bought, the APIs got limited to job titles—not necessarily all the spec that was underneath it. There is power in these pools—in particular, in profiles of jobs—because then you can go look at tasks and skills. I’d imagine there’s going to be a race here toward figuring out how we can piece these together to form the ontological cloud, if you will, of “these 17 things describe this skill.” Because it really is about skills and not credentials.
. . . And what it means for professional growth
Bryan Hancock: You can also think about this as aiding a skill-based transition not just from the employer’s perspective but from the candidate’s or employee’s perspective. In the current world, if you’re somebody who may have some skills but don’t have a very clear view of what your career opportunities might be, you are highly dependent on a manager or somebody taking an interest in you and helping to navigate you to “nontraditional” paths.
But in a world of generative AI, you could have a conversation with a very intelligent chatbot and say, “Hey, here are my skills and experiences. What jobs could be open to me?” And it could come back and say, “Well, most people with your skill profile do these things, but some do A, B, C,” with “C” being coding. And then, you could say, “Tell me what these jobs in coding would be,” and it could pull a job description for a coder that is not just geared toward an IT person but translated into words you understand. Then you could say, “OK, this is great. I’m interested. What learning experiences do I need?” And generative AI could tell you what those learning experiences are.
So for somebody who has the innate ability but not the visibility, generative AI can illuminate a range of career paths and start helping people understand how to get there.
Lareina Yee: Imagine I’m ten years into my career and I’m feeling a little stuck. What if I had a professional development AI assistant that helped me think through questions like, “What type of job should I seek? What are the types of roles within my company? How do I think about them?” and “What classes would I take?” as opposed to waiting for someone to reskill me—which sounds awful. How do I take the initiative ten years into my career to build the skill sets and understand the range of jobs available for my capabilities? That would be so cool.
Bill Schaninger: Depending on the regulatory environment you’re in, you’re not allowed to make any selection decision without a human being involved. This is particularly true in the EU. It’s a nice way of augmenting human work but not cutting out the decision making. On the employee side, it should provide much more transparency; you can actually see how close you are to a lot of things. I love it for the employee experience part. I get anxious about the selection part just because we’re still not sure about what’s in the data lake and how good people are at prompting the AI.
Lareina Yee: Right. It’s great to give you some options, but it’s not an answer or a recommendation engine. Your judgment matters.
Bryan Hancock: Another thing we’re seeing is that ChatGPT—and generative AI more broadly—can be particularly good at getting new workers more quickly up to speed.
There’s interesting research that Erik Brynjolfsson at Stanford, along with others from MIT, have recently come out with, which looks at call-center workers. They found that generative AI functionality wasn’t all that helpful for the most experienced representatives. It was incredibly helpful with new folks because they were able to get that institutional knowledge much more quickly. It was at their fingertips. They could ask a question and get the answer. So the productivity of new folks was dramatically higher. Generative AI really gets you 80–90 percent of the way to full proficiency.
Lareina Yee: Bryan, I love that, and I share the optimism.
What’s new for the performance review
Bryan Hancock: One of my personal favorite uses for generative AI on the people front is actually for performance reviews. Hear me out: I don’t want generative AI actually generating somebody’s performance review. That needs the human in the loop, needs human judgment, needs empathy.
But let me use this example of what I do as a McKinsey evaluator: I get written feedback from 15 to 20 individuals. They enter it into a digital system. I’ve got long-form feedback. I look at upward feedback scores that include written commentary as well as specific number-based scores. I look at how often people were actually deployed on engagements. I look at compliance-related measures. Did they turn in their stuff on time? A whole range of things. For me, as an evaluator, getting to a first draft is an incredibly arduous process. I take pride in the time and the thoughtfulness that goes into it.
But what if I could hit a button and get a draft? When I have each of the conversations with the 15 people that best know the person I’m evaluating, what if I had a draft I was already working from? It’s not a replacement for going through everything, but that initial synthesis would help me get more quickly to what I really need to probe for that person’s development and growth.
I’m excited about that use case because it eliminates a lot of work. At first, many people would think, “I’d never want generative AI anywhere near performance reviews.” But it’s exciting if we think of this as a productivity aid or as something that helps us be even better.
Lareina Yee: Now let’s talk about the employee he’s evaluating. The employee gets the feedback, and Bryan probably wrote it clearly, and he delivered it with empathy, so the person is feeling, “OK, I’ve got some strengths, and I’ve got some development needs.”
But what if I, as the employee, can query, “Who are five success models with my strengths and weaknesses, and what have they gone on to do? How can I visualize my career development? How can I continue to work on it?” I could also have an assistant that helps me map my professional development. In that way, when we check in a year later, I’ve really improved and increased my aspirations.
What if Bill is someone I should model myself on? Instead of Bryan having to introduce me to Bill, generative AI helps me realize that I’ve got the makings of a Bill Schaninger. I can be inspired by that. I think there’s a lot that enhances what we’ve been trying to do so laboriously for years.
Bill Schaninger: We talk about putting the manager back in performance management. Every time you talk to somebody about something good or bad, log it away. That way, at the end of the year, it’s more of an aggregation and synthesis, and it’s not a surprise to anyone. But that requires regular entry. So while I love what you’re describing, it’s not the tech that does that; it’s the people committing to the common data capture and the common approaches that enable it.
Bryan Hancock: Your point is well-taken. Then, as an evaluator, I apply my human judgment.
Bill Schaninger: The normative data is nice. When we get our sponsorship and mentorship data at McKinsey, we see how we compare to other partners in a given region. If you don’t have a reference point, though, how would you know what “good” actually is? When you get the normative data, you can start getting some guidance. I like all that, and it’s all enabled by huge amounts of data.
If this enables a more robust and wholesome view of actual performance, it makes it a whole lot easier to have a difficult performance conversation. We need to put the manager back in performance management. But can we make it easier on managers so they can spend the time managing instead of scribbling out a schedule or knitting together 15 data points?
Bias and other risks
Lucia Rahilly: Let’s talk a bit more about some of the risks. Generative AI learns based on historical data, and historical patterns of data reflect historical biases. By relying on generative-AI-driven tools, what’s the risk we are inadvertently propagating these inherited biases?
Lareina Yee: Certainly, today, generative AI can amplify bias.
Let’s say I’m recruiting, and I describe some different qualifications. I’m looking at urban centers of talent, and I decide I’d like to look for basketball captains; or perhaps, instead, I say that lacrosse captains are desirable. These are team sports with captains and leadership, so in some way that makes sense.
But if you look at demographics, who plays basketball in cities is very different from who plays lacrosse. And so, by emphasizing lacrosse, you will typically get more young White male leaders, whereas if you chose basketball, you might find more African Americans or Latinos. What about softball, where we see women? What happens if, instead, we select a whole set of sports? Even then, just the selection of the sports as a filter could amplify bias in the questioning. I think the power of the question is on us as humans.
Bryan Hancock: Of course there are also intellectual property concerns.
But I also think there’s a risk of us all becoming less interesting. If you are somebody in a creative field and you leverage generative AI to get your output up from six articles a week to 12, you’re spending less time per article. You may need to do that to get to publication in time, but that also means you’re not spending as much time in the shower, on a run, or in the car thinking about the articles. Your productivity will go up, but you may not necessarily have as much time for creative thinking. We know that the most creative thoughts come from downtime—when you’re doing something else and letting your mind wander.
This risk of being less interesting is important, and one that we may not have fully thought through yet.
Lareina Yee: Precisely. There are a lot of risks. Let’s also think about leaders who are implementing this technology. Often people had a workflow where they would think about a technology and the business return on investment, and only at the end would ask, “Are there any risks we should worry about?” I would strongly recommend that you think about risk up front in the workflow design.
The other thing is there’s a real opportunity for what we typically call “change management.” If you don’t think through how the technology changes the job, workflow, or collaboration model, then you’re not necessarily directing that additional time toward something that’s more value added. You need to think about how it affects the rest of the workday and workweek.
Bill Schaninger: In many cases, we’d like to blame the technology and not highlight the poor problem solving that happened just before implementing it. Getting a better, shinier tool that’s faster and more expansive doesn’t relieve you of the burden of thinking things through.
Lareina Yee: The bigger thing to call out here is that three of us have spent this time thinking about all the positive intentions and the ways we can use this for good. But there are probably people who are thinking about this technology and asking, “How can I use this for harm?” Traditionally, this is why government regulation, policy, and international standards play a fundamental role in our society. I don’t think you can completely leave it to the private sector to self-regulate.
Preparing for the inevitable
Lucia Rahilly: A big concern for people is that these kinds of tools will eliminate their job or—potentially even worse—become their bosses. What do you think people can do now to prepare for the changes that are coming with generative AI?
Bill Schaninger: I would try to make it easier for them to learn and play with it. This is better than continuing to try to resist it. I don’t think we should become beholden to these fears.
Lucia Rahilly: And assuming HR and talent processes become increasingly automated, how can leaders ensure that generative AI doesn’t get in the way of what Bryan called “the human in the loop?”
Lareina Yee: Leaders have a huge role to play in two ways. One is to modernize and leapfrog their own talent capabilities within their functions. And second, if 80 percent of their workforce is shifting, they play a huge role in how that happens and how it affects employees at their companies. I think leaders have a huge voice at the table.
Bryan Hancock: It’s a tremendous opportunity for HR to increase access to opportunities for huge swaths of their workforce. It’s an opportunity to get managers more consistently up to the level of performance that HR leaders have always wanted them to achieve instead of working on administrative tasks. I hope that HR would view this as an opportunity to routinize and get rid of the work that they don’t have to do. Then for the work that they do have to do, they can use this technology to find a way to get better answers more quickly.